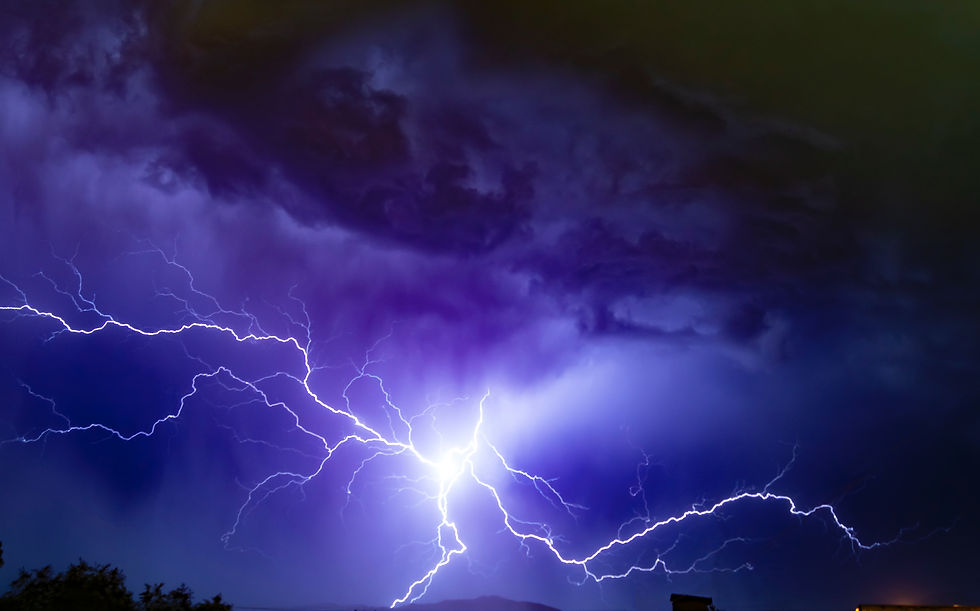
Using cutting edge cloud technology and innovative data solutions the Elastacloud team has delivered industry-leading business intelligence insights in a range of projects within the energy sector. Last year we worked with SSE, a large UK energy generation company to deliver industry-leading solutions to their data challenges.
SSE
SSE plc is primarily a developer, operator and owner of low-carbon energy assets with a strategic focus on regulated electricity networks and renewable energy. Their headquarters are in Scotland but have locations all over the UK, and they are listed on the London Stock Exchange whilst being a constituent of the FTSE 100 index. SSE also maintain and invest in transmission and distribution networks, generating and delivering electricity across the UK.
For SSE, faults along their networks, which are often caused by lightning strikes, are very costly to business operations with great impact on their customers. When blackouts occur, SSE receives costly fines from OFGEM, and as climate change continues to influence extreme weather, blackouts and the subsequent fines are likely to increase. SSE´s goal was to protect their customers in the areas with the highest chance of lightning strikes, and to do so they had to identify the top 100 locations that have the highest risk of faults and the greatest impact on customers when those faults occur. Additionally, with these insights they can better and more cost-effectively install surge resisters to reduce risk of faults from lightning strikes.
SSE consulted Elastacloud because they wanted to build a Machine Learning model that would target these high-risk areas using historical and current data. They wanted an interactive dashboard platform that would help them make better business and operational decisions.
The Data
SSE collect large amounts of geographical, meteorological, engineering and network data to understand the key features of their locations such as weather patterns and customer demographics. The potential of these large data sets a yet untapped and could be used to better predict and respond to faults. The Elastacloud data science team collected these data sets and explored correlations between them.
The first step was to join the data sets to each other by connecting the geological, meteorological, customer and faults data. For instance, the lightning strikes were compared to the faults data to identify if lightning was a potential cause. The team were then able to combine geographical data and frequency data to show the areas of most probable lightning strikes, which included analysing engineering and land usage data to discover which areas were most susceptible to faults.
Using this data the team were able to build machine learning models to find the lightning strike hotspots.
A Machine Learning Answer
The Elastacloud team used machine learning algorithms to build AI models that used the historic ´training data´ to make predictions or decisions for future faults involving lightning strikes on a given task. The models consider a variety of features of the lightning strikes such as distance, duration and frequency. Adjoining this to probability data, information about the cause of a fault and the likeness of it occurring can be seen with great accuracy.
With complex data sets like this, the presentation needs to be clear. Our BI Team used SQL and Power BI capabilities to structure and categorise the features of the data into multiple segments. The platform created from these tools gave SSE the ability to view the dates, equipment identification numbers and regions in a single comprehensive report with an intuitive and interactive front end. The report gave SSE insight into the following features of the faults:
· Time occurred
· Cause
· Location
· Frequency
· Consumer impact
· Costs
The models provide SSE with cutting edge insights around power blackouts and surges in SSE’s generation and identifies the top 100 hotspot areas along their networks. This allows SSE to make smarter, more targeted and cost-efficient decisions when implementing surge resistors using the machine learning models as a validation tool. Through this, SSE is better equipped to reduce the number and duration of electricity blackouts foregoing heavy fines from OFGEM and improving electricity supply efficiency for their customers.
Find out more about Elastacloud in the Energy Sector here.
Or if you want to read more stories of our projects and customer experiences click here.