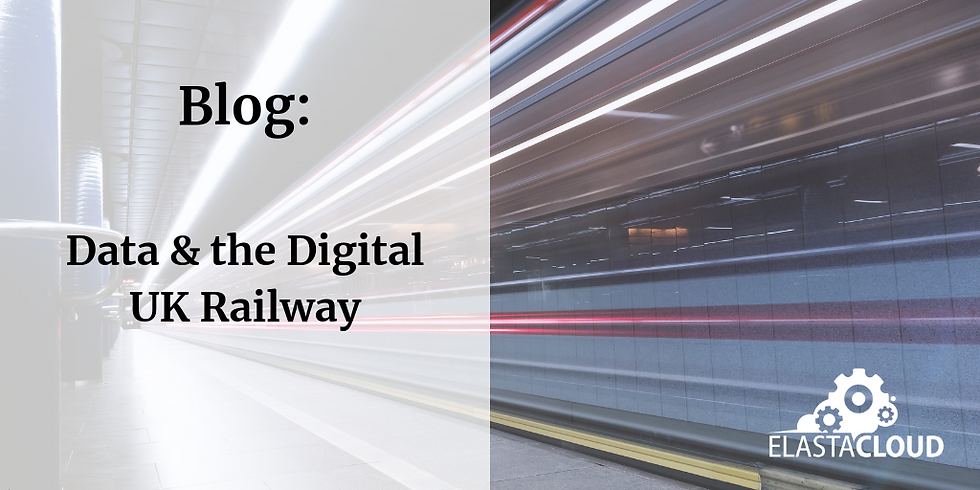
Rail companies use data analytics daily to run everything from operations and maintenance to making key business decisions based on intelligence from the collected data. In the last 10 years, the emergence of the digital railway has incurred a demand for Big Data techniques to respond to an overwhelming surge in data collection capabilities and speed.
Largely, the UK rail sector has embraced this development and has begun implementing Big Data analysis and Data Science technologies to stay ahead of competitors and provide a better modern service to their customers while maintaining assets and efficiency.
The Digitisation of Rail
Historically, the rail industry has been criticised for lack of innovation, falling behind the times in many areas and failing to capitalise on emerging technologies. Certainly, this has been improving in recent years. Today, companies collect vast amounts of data from rail stakeholders who provide intelligence via computer systems, the Internet of Things (IoT) and Cloud computing, which constitutes the move towards ‘smart railways´.
To ensure the data’s business potential is realised and optimised, companies analyse and work to unlock the potential of the collected data, as Porterbrook, the train leasing company have done in the last year. The digitalisation of rail also offers new services for customers. Mobile ticketing has undoubtedly revolutionised the travel experience and new technologies such as the Trainline´s voice activated customer communication greatly enhances the way in which customers interact with companies. Undoubtedly, digital technology governs a great deal of rail customers behaviours including purchasing, expectations, operator information and reservations.
These leaps in digitisation in rail have led to improved monitoring of assets, automation in operations, and customer interactions, and as the technology becomes readily available and cheaper, the digital railway nears reality.
Where Has the UK Rail Industry Been failing?
While digitisation has provided the means for innovation and improvement of operations, recent reports indicate performance of trains services have not met expectations. Since the privatisation of Britain’s Rail Network, the government predicted that more competition between the companies in the sector would lead to a better service. In fact, the opposite has been true as ticket prices have risen, and lateness hit a 13 year high in the UK in 2018.
The issue is that while UK rail has certainly improved the maintenance and delivery of rolling stock assets, it is still way behind other countries in customer experience. Customers simply do not trust rail companies to deliver on time, and why should they given recent statistics? According to the Office of Rail and Road, 86.3% of trains arrived on time in the UK. In comparison, the Spanish high-speed network achieves 98.5% punctuality. Dan Ascher´s BBC article suggests that future rail companies should be underpinned by punctuality and excellent customer experience.
Many argue the root of this problem is a reluctancy to invest in smart technology combined with a lack of data science workers within rail companies. Indeed, it will require a change of mindsets and business models as well as significant financial investment in rail digitisation to improve operations and rekindle customer faith. Improvements will also need to be made into customer interactions utilising new technology to provide up to date, accurate and accessible information for passengers.
How Can Data Science Improve the Rail Industry?
Data Scientists extract meaning from and interpret large quantities of data. In rail, this includes all the current and emerging data used by railways to help monitor infrastructure and equipment and optimise maintenance to improve safety.
“Big data analytics have the potential to influence several dimensions of the railway sector and can overcome organisational, operational and technical complexities, including economic and human effects and information handling.”
- Professors D. Galar, U. Kumar and R. Karim at Luleå University of Technology.
Operations and maintenance are areas generating considerable excitement for rail companies due to self-learning and smart systems that predict failure, make diagnoses and trigger maintenance actions. Companies looking to introduce smart technology systems require data scientists to build these predictive Machine Learning (ML) models and provide a service that helps uncover the potential of the data.
ML models and data science services that predict delays across the network are popular because they can:
Help make savings on delay-repay compensation
Identify interventions to minimise disruption
Inform better train scheduling
Inform better maintenance scheduling
Improve public performance metrics
To utilise the insights from these models, the right platforms needs to be well utilised for a company's specific data sets and requirements. Data scientists and rail industry experts should seek to collaborate to build the necessary algorithms and analysis tools to deliver the most effective solutions for the business' needs.
The UK rail leasing company Porterbrook recently consulted Elastacloud to realise these advantages.
Read the full case study here.
Follow us: